Senvol Analysis Software Reduces Trial and Error of Additive Manufacturing Processes
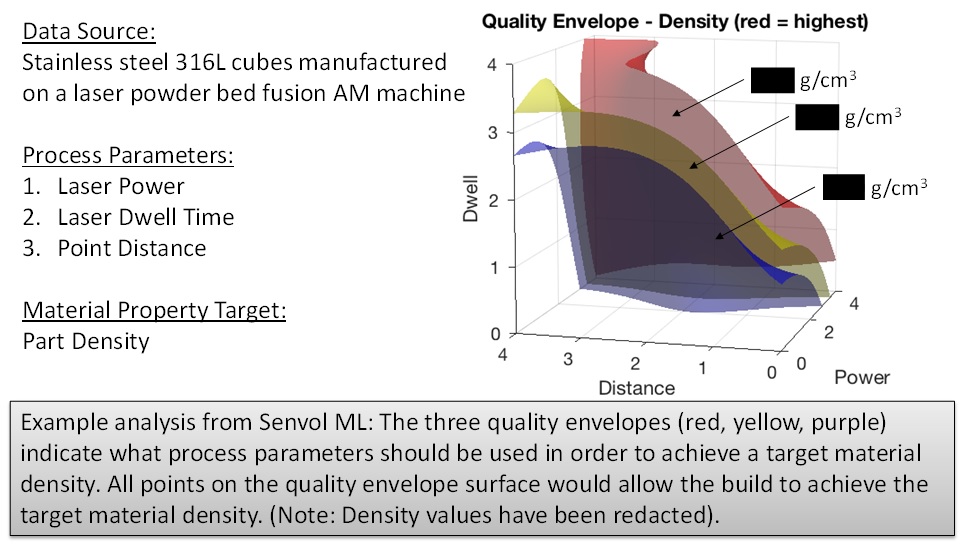
Example of Senvol new machine-learning software for optimizing additive manufacturing process parameters. Users can work with many possible desired outcomes and/or input parameters. Image courtesy Senvol.
Latest News
March 29, 2018
After less than five years of operation, Senvol of New York City has become the go-to company for nitty-gritty data behind additive manufacturing.

Now in its second phase of funding from the U. S. Navy’s Office of Naval Research, the Senvol ML project is aimed at reducing the trial-and-error approach to defining AM material-process procedures. Zach Simkin, co-president of Senvol, says the company has developed a proprietary algorithm that can be “trained” to predict an unlimited variety of performance goals.
“Any material property or mechanical performance target can be addressed,” explains Simkin, “such as density, surface roughness or fatigue life. Because our software is data-driven, we can analyze just about any variable as input and/or output.”
Data Plus Analysis Yields Desired Properties
One of the many beauties of this software tool is the fact that the math behind the algorithm is material/machine/process agnostic. For input, users quantify the desired outcome, such as a final material density of 8.4 g/cm3 then name the input parameters that can be varied. For a chosen AM system, the algorithm already includes default, optimized process parameters. The power of Senvol ML is that the algorithm has already “learned” and continues to learn from previously input datasets.For example, the analysis result for a powder-bed system could show the range of values for laser power, laser dwell time and point distance that, in any combination, would create parts with that density. The reason for a range (instead of a point), presented as a 3D graphical envelope of possible settings, is because there are trade-offs to be made among the parameters (e.g. high laser power must be compensated with high scan speed so that a user is not putting too much energy into the powder bed) – and users need to work in the real world.
“The Senvol ML algorithm also has the capability of showing the AM user which parameters are sensitive and which are robust based on where they’re located in the dimensional space,” says Annie Wang, co-president of Senvol. “Users might choose to run the system with a faster scan speed, to shorten print time, if they know they can increase the laser power and still end up with a part of the desired density.”
Moving Ahead with Machine Learning
Senvol’s proprietary algorithm has been developed specifically for AM applications; it uses either empirical or simulated data and can be applied to any AM process, any AM machine and any AM material. The work has been validated across multiple datasets during the Phase I Base and Option periods of the company’s Small Business Technology Transfer (STTR) project with the Navy.Eventually the Senvol ML software will include the following four capabilities:
- Forward Prediction – predicting mechanical performance (such as fatigue life) from a given set of process parameters
- Inversion – given a target value (e.g., desired tensile strength), the algorithm will determine what process parameters to use
- Machine Learning – continuing to learn from previous datasets and applying that knowledge to new datasets, improving output accuracy and reducing the amount of needed data for new builds
- Recommended data collection – suggesting to users just which data points are needed to improve prediction accuracy, again saving time and effort
In both cases, he explains, the tools analyze data from each layer of the build. Users can then correlate relationships between irregularities in the build and the resulting mechanical performance.
The company will be demonstrating use cases and actual results during presentations at the upcoming AMUG 2018, RAPID +TCT 2018 and CAASE18 conferences.
With the announcement of Phase II STTR funding, Senvol says its software will be made commercially available to any company looking to qualify AM parts. Contact Senvol ([email protected]) for more details, especially if you are interested in the beta-stage program.
Subscribe to our FREE magazine,
FREE email newsletters or both!Latest News
About the Author

DE’s editors contribute news and new product announcements to Digital Engineering.
Press releases may be sent to them via [email protected].
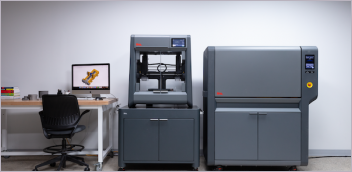
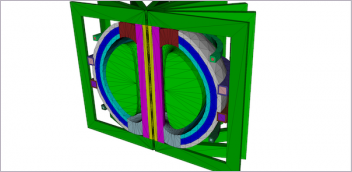
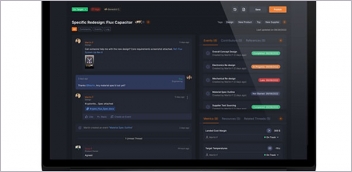
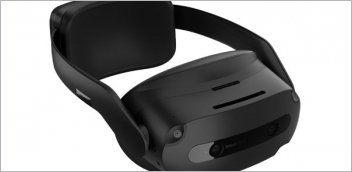